Automating processes just because it is fancy or just because the solution approach is innovative won’t generate any business value. As data is one of the most valuable assets a company can have, automation must be a strategic solution based on the goal of using data more efficiently. According to a study published by Seagate, the amount of generated and available data within a company is nearly doubling every year between 2020 and 2022. In this sense, this blog sheds a light on the major challenges for turning data into value by simultaneously pointing out the key role Robotic Process Automation (RPA) is going to play.
Challenges for turning data into value
There are two dimensions when looking at turning data into value. First, it is about minimizing workloads and error rates of data used within operational and transactional processes. This is important to ensure high data quality and to make human resources available for more value-adding tasks. Second, it is about identifying, collecting, validating and structuring data to gain more objective insights through analyses. This enables businesses to work at a faster pace and to make critical business decisions based on facts rather than on suggestions. But while of turning data into value, companies regularly face multiple challenges. Figure 1 lists the main challenges.

Where Robotic Process Automation can play a key role
Through the adoption of RPA, much more data can be collected faster, also ensuring a higher data quality compared to the traditional manual processes. Whereas human employees regularly spend a tremendous amount of time trying to identify, collect, clean, structure and enhance raw data for better decision-making, RPA can collect and feed the data directly to analytic tools or machine learning algorithms. In this way, RPA in combination with other tools can provide you with the data to make more objective decisions – not biased by personal views or relationships. This is an immense competitive advantage in times where the amount of generated and available data is increasing exponentially. Figure 2 highlights where the adoption of RPA can play a key role for overcoming the described challenges.
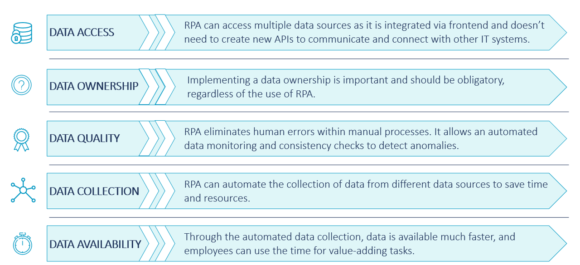
Example use case for data collection: web scraping
The web is full of information which can be helpful to get time-bound insights and derive beneficial decisions. But browsing through the web manually can be a very labor-intensive task. Let’s take for example a company that is dealing with real estates. Its goal is to buy rentals that meet specific criteria for a low price in order to rent or resell it at an appropriate time to make profit. The traditional approach would be to browse daily through a given set of websites to detect good offers. Let’s assume that this takes about two hours – every day. By applying RPA, the bot automatically browses through the different websites and checks if the given criteria are met (e.g., distinct region or minimum number of rooms). If the criteria are met, the bot finally checks if the price lies within a range which was predefined as “profitable”. If this is the case, the bot saves the offer in a list and automatically informs the human employee via e-mail.
As possible extension to this use case, RPA could also be used to collect and feed the offers directly from the different websites to a machine learning algorithm which analyses if it is a good offer or not, based on historical data.
Processes are the pipelines, data the transported good
RPA is about the automation of processes. These processes are the pipelines which transport the necessary data to generate value. The fact that more and more data is available every day is a challenge but more important it is a fundamental chance to get more information through analysis – information which can make a difference. In order to handle the increasing amount of data and to enable a faster time-to-value of analytics projects, more and more enterprises migrate their data to the cloud using data lakes or data warehouses. As the cloud is clearly the future, it is important to have a data strategy in place and to build the processes and automations around it – and not vice versa.
This blog is part of a series:
The first blogpost introduced four key pillars for the long-term success of an automation project: Robotic Process Automation I: A Path of Hyperautomation
The second article focusses on the importance of having a process perspective and shows three steps to select the most profitable processes: Robotic Process Automation II: How to Select the Most Profitable Processes