Planning for a global supply network at a tactical level can be challenging, especially when creating production plans that need to consider varied, interchangeable components across a global supply network. How can supply planners account for all constraints of a wide array of components to create a feasible production plan while maintaining inventory levels? This problem can get even more challenging when a supply network faces constraints in production capacity and component-specific requirements due to changing regulations. This is especially common in the pharmaceutical industry, where these alternative components are called flavors.
A supply planning optimizer offers powerful tools for production planning in a tactical planning horizon. The optimizer algorithm can calculate an optimized supply plan while considering constraints for alternate components, which is especially relevant for pharmaceutical supply planners who need accurate production plans for flavors. This article describes how we leveraged the capabilities of a market-leading supply planning optimizer to effectively address the flavor management problem.
The Flavor Management Problem in Supply Planning
To fully understand the added value of a supply optimizer for supply planners, we must first discuss the flavor management problem in depth. As mentioned, a flavor in a pharmaceutical supply network represents an interchangeable component that is required for finished good production. The component itself can exist at any level in a production sequence. Due to the strict requirement to comply with regulatory approval processes, these components must be designated as different stock keeping units (SKU) based on their production country of origin and resource technology despite being the same physical and chemical product, and hence we have flavors.
In tactical supply planning with flavors, country-based restrictions at the receiving sites must be accounted for. A supply planner needs a production plan that reflects these restrictions by only sourcing flavors from countries or regions specified by regulation and that can also adapt to changing regulations. In addition to region-specific regulation, flavors also increase the complexity of production sourcing. If a singular finished good has ten possible flavors that can be used to fulfill a production order, how does the supply planner know the optimal mix of flavors to supply for finished good production? If a finished good has a preferred flavor for production and the resources used to produce this flavor experience an unexpected shutdown, what alternates should the supply planner select to fulfill production on time? Supply planners need a tool that can model alternate flavors and calculate worst-case scenario production plans.
Mastering Challenges with the Supply Optimizer
Through the precise configuration of the relevant master data types and the input key figures of the SAP IBP Supply Optimizer, we were able to utilize its full capabilities to solve the challenges of flavor management. By translating alternate bill of materials (BOM) to flavors in our data model, we were able to create a supply network that accounts for all possible flavors that a finished good might have in its production plan. In addition to the custom configuration of the master data, we tailored the priority inputs of the optimizer so that each possible flavor would be considered in production planning. With this expert configuration in place, we were able to leverage the optimizer’s planning algorithm to create optimized production plans. This allowed us to consider all flavors as production inputs while supplying external demands and meeting inventory targets.
When a supply optimizer planning run executes, it propagates forecast demand and inventory targets from downstream locations to the upstream manufacturing sites. In our implementation, a distribution center was the most downstream location. The quantity of the forecast demand and inventory for a finished good would then be communicated to the finished good manufacturing sites, heretofore known as finished good (FG) manufacturing. From the FG manufacturing sites, the amount of FG SKUs needed for production would then propagate demand to the semi-finished good (SFG) manufacturing sites for the different flavors needed in FG production.

With this demand propagated across the supply network, the optimizer then uses the available production resources to supply the requested demand. In our model, the demand for a single FG SKU could be fulfilled by several different SFG SKUs (flavors). Using the different input key figures, in particular the production and transportation priority inputs, we were able to create a prioritized hierarchy of flavors. For example, a finished good with an SKU of FG1 could use any semi-finished good from a selection of SKUs SFG1, SFG2, SFG3, and SFG4 for production, but planners prefer to use the flavor 1 SKU (SFG1). By increasing the production priority via the optimizer inputs for the flavor 1 SKU relative to flavors 2-4, planners can instruct the optimizer to prioritize production of flavor 1 relative to the other flavors when planning production for FG1.
To continue our example, each of the above four flavors is produced by separate resources at different SFG manufacturing sites. If the resource used to produce SFG1 experiences an unexpected capacity shutdown, planners will now need SFG2, SFG3, and SFG4 supplied as alternates to the FG manufacturing sites so that forecasted demands can still be met on time. The optimizer would then automatically calculate the correct mix of flavor alternates to supply using the priority inputs specified by the planners while considering the available capacity of the alternate flavor resources and the transportation lead times from the other SFG manufacturing sites.
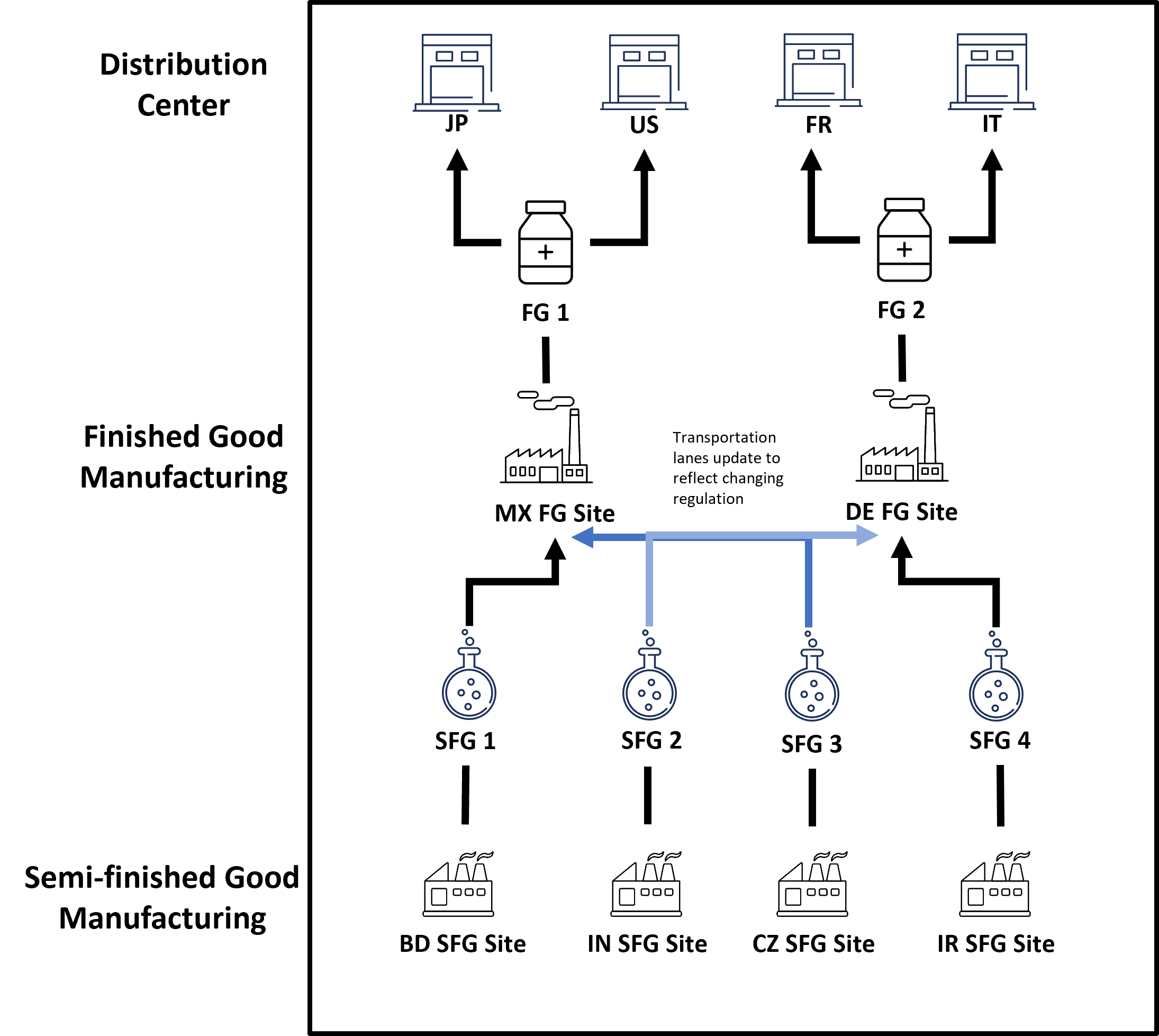
Lastly in solving the regulations challenge, planners can leverage the optimizer data model to restrict the supply of flavors from country-specific SFG manufacturing sites to receiving FG manufacturing sites. By tailoring the transportation lanes of each SFG, planners can restrict the transport of certain flavors based on their regional origin. The optimizer will then utilize the transportation lanes that are available to each FG manufacturing site to supply the regulation-approved flavors in the production plan. Additionally, if regulations change, the supply planners can then update the transportation lanes to account for new restrictions, and the optimizer will then calculate a new production plan accordingly.

Having a system that automates flavor selection for a tactical supply plan offers immense value for pharmaceutical supply planners. In addition to saving planners significant amounts of time in the planning process, the optimizer removes the guess work in determining the optimal selection of flavors to use in production. This removal of guess work leads to increased accuracy in the tactical supply planning process, which reduces inefficiencies when translating this supply plan to the operational level.
Conclusion
The flavor management problem presents a very real challenge to production planners in the pharmaceutical industry. A production plan needs to consider all regulatory restrictions for a wide variety of alternate components while understanding the optimal mix of these components. In answering this problem, supply optimizers are the suitable planning tool to deliver tactical production plans while considering these complex constraints. In addition to improving planning at the tactical level, the supply optimizer can also enhance operational execution by delivering the most accurate tactical parameters to operational planners.
Through the configuration of the underlying data model, supply planners in the pharmaceutical industry can leverage this tool to accurately solve the flavor problem and greatly improve their planning processes. In an age where maximizing efficiency and accuracy in your production plan is critical, the supply optimizer provides the necessary toolset for supply planners to achieve planning excellence.